From AI Critic to AI Fanboy
Some years ago, I could no longer stomach the hype surrounding AI that it never lived up to. I left the field after finishing my master’s degree and went on to get my PhD in human creativity in the field of cognitive psychology.
Then came large language models (LLMs), particularly ChatGPT, and the field was finally beginning to live up to the hype that had been promised since the term AI was first coined in 1956 by John McCarthy.
All my psychology research on improving human creativity was now ready to pay off in a big way. ChatGPT had the same creative blindspots as humans do because it was trained on human-generated text. The human obstacles to creativity are implicit in the text humans produced. Consequently, the same techniques that helped humans be more creative also worked to make ChatGPT more creative. Better than that, ChatGPT followed my creativity algorithms more closely than humans ever did, so they had a maximal effect on ChatGPT’s improvement.
I now knew how to build an extension to ChatGPT that made it into a highly creative problem solver. My path was coming full circle. I started in AI, left it to study human creativity, and now everything I developed for humans also worked to make ChatGPT incredibly creative.
For example, ChatGPT (and GPT-4) failed to solve any of the creativity problems I give to human participants. It went zero for 12 (0%) for my 12 favorite creativity problems. College students averaged about 37% success rate on these problems when given no special training. So, the average college student was much better than ChatGPT on these creativity problems. When college students were trained on my techniques, their performance improved to about 62%. Because ChatGPT executed my techniques more faithfully than the college students ever did, its performance soared to 100% (12 for 12).
Here are a couple examples of the creativity problems: (1) How can you save everyone on the Titanic? (2) I need a rental car by day’s end for a short trip and none are available. What should I do?
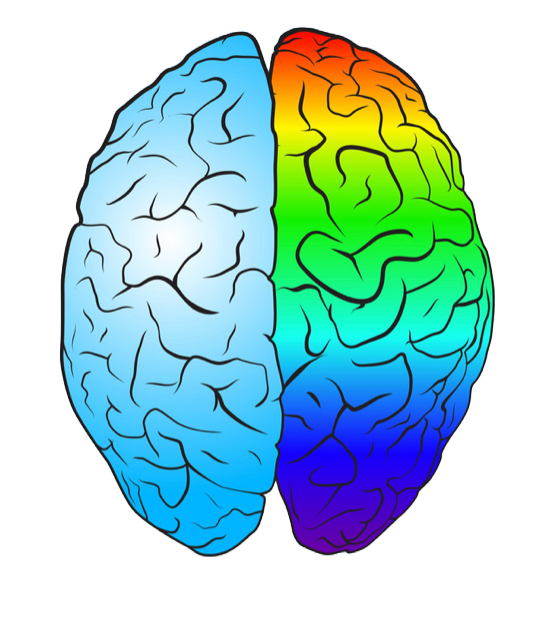
Both humans and ChatGPT suffer from “functional fixedness,” which is the tendency to fixate on the designed use of an object, and not on the physical properties of the object itself. In the Titanic problem, people tend to fixate on the lifeboats as the things to save people. However, lifeboats are just one of many things that float. There are many other things that float on the ship: wooden tables, wooden doors, planks, steamer trunks, and car tires from the many cars on board. These items could be used to make standalone floating surfaces or surfaces that bridge the area between the lifeboats, thus expanding their capacity. Further, the iceberg itself is a large floating surface that could have possibly kept many people out of the water until help arrived. Ice floes had been used in other ship disasters to save passengers’ lives.
Regarding the rental car problem, ChatGPT and humans tend to fixate on solutions with cars, even though none are available. This fixation is called “goal fixedness” because people stay very close to the original wording of the problem. A simple solution is to rent a U-Haul van for $19.95 a day plus fuel and mileage, which, for short trips, is much cheaper than any rental car. The necessary reframing for these problems takes place by simply re-describing the crucial objects generically: lifeboat to floating thing and rental car to rental vehicle.
Functional fixedness and goal fixedness are just two mental obstacles to creativity. There are many others and for each one, I have an effective technique that my extension to ChatGPT now uses.
Importantly, ChatGPT does have areas of creativity where it is better than humans. For example, if given a particular keyword, such as “candle,” my human participants that work hard can list perhaps 25-30 items that candle reminds them of: wick, flame, birthday cake, wax, romance, power outage, candle holder, many colors, many scents, etc. A participant that does not work hard will only come up with maybe six-12 items. ChatGPT can easily come up with 100 to 150 items. How does this ability help with creativity?
Certain creativity tasks require the connecting or blending of two or more concepts. For example, how many possible meanings could you create for the noun-noun combination “steak puddle?” Humans tend to come up with three or four, but ChatGPT can easily create two dozen or more. A steak puddle might be the puddle of liquid that forms from a defrosting steak. Or, a sudden, heavy rainfall during an outdoor BBQ turns the steak on the grill into a steak puddle. Or, a toothless man still loves steak so he puts his cooked steak into a blender to produce a steak puddle. Because ChatGPT might have 100 to 150 associations for both steak and puddle, it has many more avenues available than humans do to combine the two words.
In brief, for any creativity task that requires combining or blending together two or more concepts into a coherent unity, ChatGPT easily outperforms humans. This finding explains why AI image generation, when it is done well as with Midjourney, is judged by people to be much more creative than text generation (as with GPT-4). The prompts for image generation mostly involve combining two or more concepts. For example, the prompts “produce an image that combines an elephant” and “a butterfly or paint a server farm in an impressionistic style.” Both explicitly mention what should be combined or blended together. In contrast, many writing prompts do not require combining or blending (e.g., write an essay on Hans Christian Andersen).
The wait has been worth it! All of my creativity research now directly applies to enhancing the creative abilities of ChatGPT, and it is a very good student.
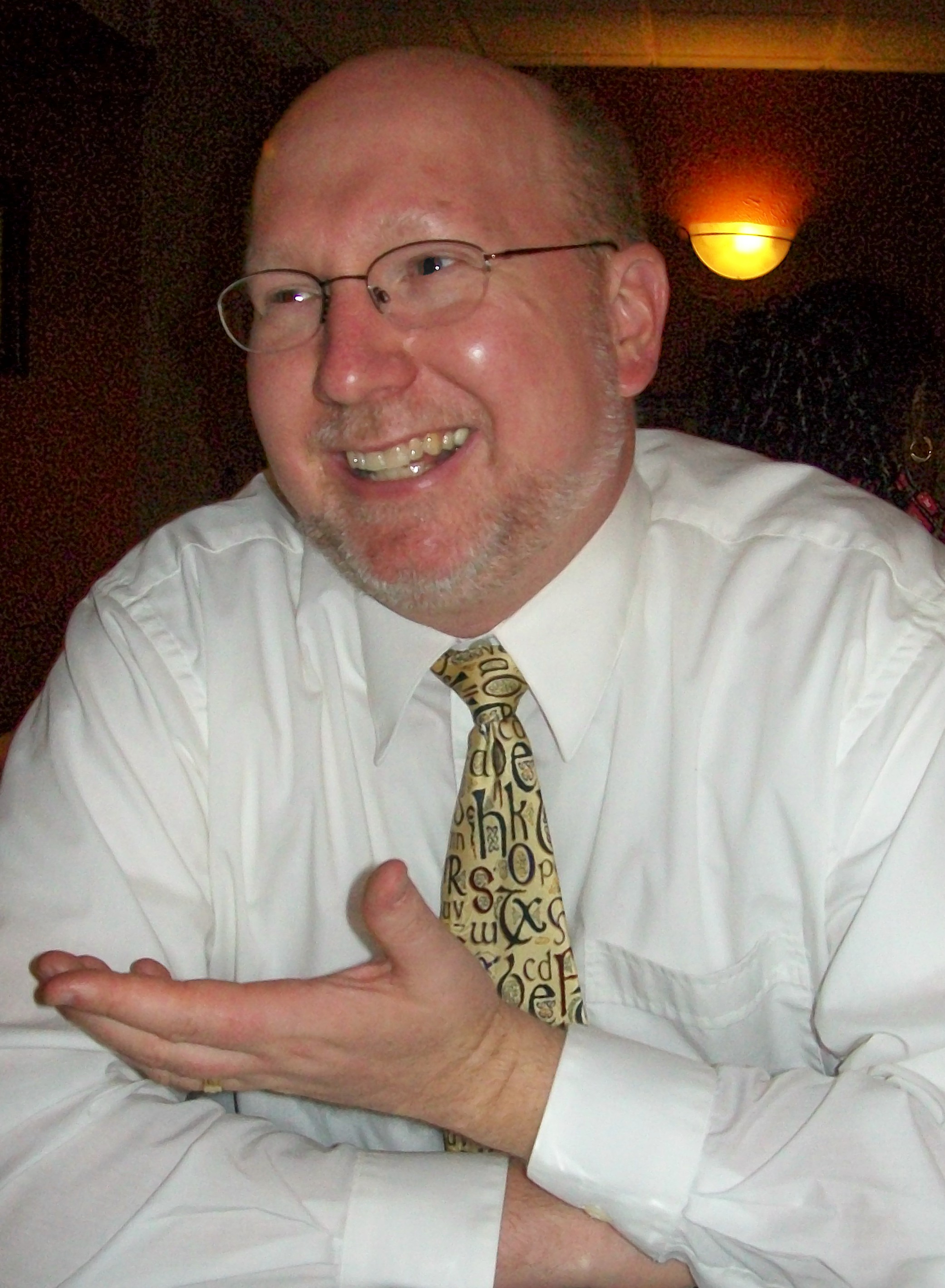
Edited by Lauren Lombardi
McCaffrey, T. & Pearson, J. (December 2015). Find innovation where you least expect it.
Harvard Business Review, 82-89.
https://hbr.org/2015/12/find-innovation-where-you-least-expect-it
Harvard Business Review (Producer) (2015). Why we can’t see solutions in plain sight [Video].
https://hbr.org/video/4653517227001/why-we-cant-see-solutions-in-plain-sight
Harvard Business Review (Producer) & McCaffrey, T. (Writer) (2014). BrainSwarming: Because brainstorming doesn’t work [Video].
http://hbr.org/video/3373616535001/brainswarming-because-brainstorming-doesnt-work